Importance of Data Quality in AI
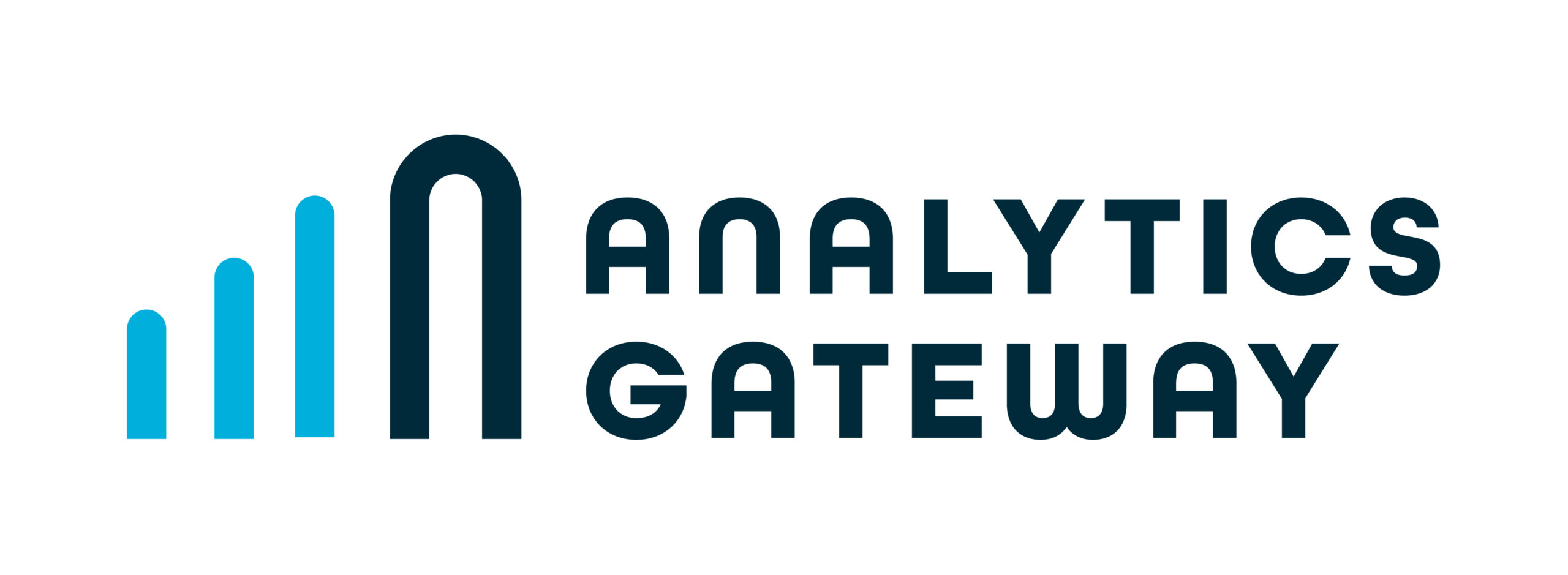
Accuracy of AI Models
The accuracy of Artificial Intelligence (AI) and Machine Learning (ML) models depends significantly on the quality of the data used for training. High-quality data, which is accurate, complete, and representative, leads to more reliable and robust models. Conversely, poor-quality data can result in models that make inaccurate predictions or exhibit biased behavior.
Bias and Fairness
The quality of data also impacts the fairness of AI models. Biased or unrepresentative data can lead to models that are unfair to certain groups. For example, if a dataset used to train a facial recognition system predominantly contains images of people from certain racial backgrounds, the system might perform poorly for individuals from underrepresented groups. Ensuring data quality includes addressing biases to create more equitable AI systems.
Confidence in Decision Making
AI models are increasingly used to support or automate decision-making processes in critical areas such as healthcare, finance, and public safety. The decisions made by these models can have significant implications. High-quality data ensures that the models’ decisions are based on accurate and relevant information, which is essential for building trust and confidence in AI-driven systems.
Efficiency and Cost-Effectiveness
Poor data quality can lead to inefficiencies in the AI development process. Models trained on low-quality data may require more iterations of training and fine-tuning, consuming more time and computational resources. This not only increases the cost of AI projects but can also delay the deployment of AI solutions.
Compliance and Ethical Considerations
With the growing adoption of AI, there are increasing legal and ethical considerations around the use of data. High-quality data that adheres to privacy regulations and ethical guidelines is crucial for compliance. Poor data practices can lead to violations of laws and ethical standards, resulting in legal penalties and damage to an organization’s reputation.
Generalization and Scalability
For AI models to be effective in real-world applications, they must be able to generalize from the data they were trained on to new, unseen data. The quality of data, in terms of its diversity and representativeness, plays a crucial role in a model’s ability to generalize effectively. High-quality data ensures that AI systems can be scaled and applied to a wide range of scenarios.